Data Science vs Machine Learning vs Artificial Intelligence
Deep learning is used for many applications in the real world, such as customer relationship management, mobile advertising, image restoration, financial fraud detection, and natural language processing. The system is autonomous and learns from itself, requiring minimal human intelligence to continue self-improvement. By clustering data together, the model constructs patterns which it then uses to identify future examples. Any examples are added to the cluster of data collected by the model. It involves training an algorithm, often referred to as a machine learning model, with a large dataset. The model looks for patterns in the dataset, which has both a ‘cause’ and an ‘effect’ variable attached to each entry.
Did this article help you understand the difference between AI, ML, and DL? Let us know on LinkedInOpens a new window , TwitterOpens a new window , or FacebookOpens a new window . Check our ‘How to Use the Advantages of Machine Learning’ for more details, benefits, and use cases. One of the best examples of AI appliance is self-driving cars and robots. You have probably heard of Deep Blue, the first computer to defeat a human in chess. Deep Blue could generate and evaluate about 200 million chess positions per second.
AI vs Machine Learning vs Deep Learning: Applications
Unlike web development and software development, AI is quite a new field and therefore lacks many use-cases which make it difficult for many organizations to invest money in AI-based projects. In other words, there are comparatively fewer data scientists who can make others believe in the power of AI. There are two ways of incorporating intelligence in artificial things i.e., to achieve artificial intelligence.
- The Master of Data Science at Rice University is a great way to enhance your engineering skills and prepare you for a professional data science career in machine learning or AI.
- With AI being considered a general term for any type of technology that mimics or exceeds human intelligence, ML and DL are powerful ways to apply this technology toward your business goals.
- And people often use them interchangeably to describe an intelligent software or system.
This type of machine learning involves training the computer to gain knowledge similar to humans, which means learning about basic concepts and then understanding abstract and more complex ideas. Machine Learning is a branch of Artificial Intelligence and computer science that uses data and algorithms to mimic human learning, steadily improving its accuracy over time. At IBM we are combining the power of machine learning and artificial intelligence in our new studio for foundation models, generative AI and machine learning, watsonx.ai. An increasing number of businesses, about 35% globally, are using AI, and another 42% are exploring the technology.
AI vs Machine Learning vs Deep Learning: How They Work?
This is then presented on the main page of the platform for the user to choose from under the label ‘Recommended for You’. It consists of methods that allow computers to draw conclusions from data and improve with experience. Data science involves analysis, visualization, and prediction; it uses different statistical techniques. This is the piece of content everybody usually expects when reading about AI. Foundry for AI by Rackspace (FAIR™) is a groundbreaking global practice dedicated to accelerating the secure, responsible, and sustainable adoption of generative AI solutions across industries.
Varonis Systems (VRNS) Q3 2023 Earnings Call Transcript – The Motley Fool
Varonis Systems (VRNS) Q3 2023 Earnings Call Transcript.
Posted: Tue, 31 Oct 2023 03:00:19 GMT [source]
To learn more about AI, let’s see some examples of artificial intelligence in action. Artificial Intelligence comprises two words “Artificial” and “Intelligence”. Artificial refers to something which is made by humans or a non-natural thing and Intelligence means the ability to understand or think. There is a misconception that Artificial Intelligence is a system, but it is not a system. High power GPUs are the most essential requirement for Machine Learning or Deep Learning.
Difference Between Machine Learning (ML) and Deep Learning (DL)
Traditional programming similarly requires creating detailed instructions for the computer to follow. A 12-month program focused on applying the tools of modern data science, optimization and machine learning to solve real-world business problems. Both artificial intelligence and machine learning can help keep global supply chain networks functioning, even as they grow more complex, with more vendors all the time.
While creating an AI system that is generally as intelligent as humans remains a dream, ML already allows the computer to outperform us in computations, pattern recognition, and anomaly detection. Read more materials about ML algorithms, DL approaches and AI trends in our blog. DL comes really close to what many people imagine when hearing the words “artificial intelligence”. Programmers love DL though, because it can be applied to a variety of tasks. However, there are other approaches to ML that we are going to discuss right now.
The HubSpot Customer Platform
Likewise, these tasks include actions such as thinking, reasoning, learning from experience, and most importantly, making decisions. As such, AI aims to build computer systems that mimic human intelligence. The term “Artificial Intelligence”, thus, refers to the ability of a computer or a machine to imitate intelligent behavior and perform human-like tasks.
By doing so, machines are able to make predictions with minimum human intervention. For example, ML allows computers to recognize pictures of different people. Another benefit of AI is its ability to learn and adapt to new situations. ML algorithms can train machines to recognise patterns and make predictions based on data, enabling them to learn from experience and adapt to changing circumstances.
Data scientists who work in machine learning make it possible for machines to learn from data and generate accurate results. In machine learning, the focus is on enabling machines to easily analyze large sets of data and make correct decisions with minimal human intervention. Skills required include statistics, probability, data modeling, mathematics, and natural language processing.
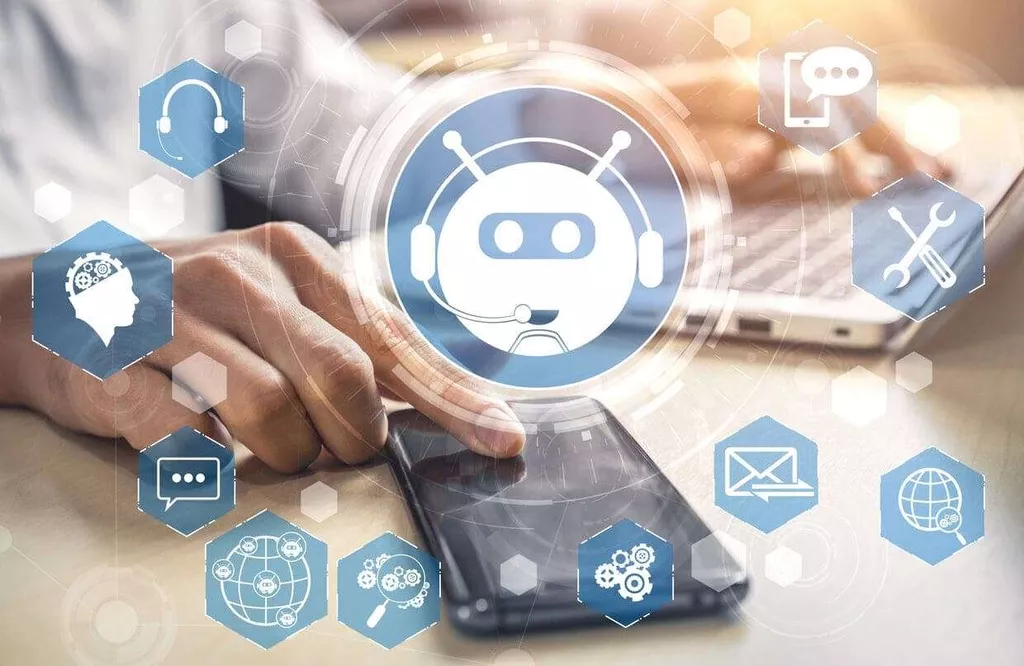
Read more about https://www.metadialog.com/ here.